Qualified scale-down models of large-scale cell culture processes are essential to conducting studies for applications such as investigating manufacturing deviations, enhancing process understanding, and improving process robustness. For example, scale-down models can be used for raw material investigations as well as evaluation and qualification of new good manufacturing practice (GMP) cell banks for manufacturing implementation. Process characterization studies are performed also with qualified scale-down models to improve process consistency (1, 2). Often it is impractical to conduct investigational studies at large scale; therefore, results gathered from a qualified scale-down model are valuable as predictors of at-scale performance.
One important consideration in developing a small-scale model is to identify which parameters depend on or are independent of scale. Scale-dependent variables are adjusted to accommodate for scale differences such as vessel geometry so that process profiles will be comparable with those at large scale. Scale-independent variables such as temperature and pH usually can be matched to those at large scale without adjustment. Agitation and gas transfer rates are two scale-dependent variables that must be evaluated to provide comparable mixing and mass transfer between two scales when feasible (3–6).
Typical approaches to scaling down agitation rate are based on power:volume-input (P/V) or tip-speed matches with those at large scale (3, 6). Computational fluid dynamics (CFD) also can be applied toward understanding the mixing characteristics of different agitation rates (7–10). Studies that evaluate and measure the oxygen mass-transfer coefficient (kLa) are important to understanding the gas-transfer characteristics of a scale-down model system in comparison with the full scale version (11–12). The oxygen-sparger configuration in relation to sparging of other gases such as nitrogen for CO2 removal, sparger element type, and pore size also should be explored to determine an optimal design that yields efficient mass transfer of oxygen and CO2 removal, which can result in comparable performance to that of the large scale.
A scale-down model is qualified to demonstrate its equivalent performance with that of the large-scale process and to increase confidence in data generated by the scale-down model. To qualify such a model, process engineers commonly use the statistical two one-sided test (TOST) to demonstrate equivalency (13–14). Data points for key process parameters that represent growth, metabolism, and productivity are evaluated with TOST to determine whether the model and large-scale processes are statistically equivalent. In a TOST analysis, the null hypothesis states that the difference between two group means is equal to or greater than a predefined tolerability limit. If statistical evidence is strong, the null hypothesis is rejected, and the two groups are determined to be statistically equivalent (15).
When nonequivalency is observed for a particular data point, justification for the nonequivalency is investigated first to identify potential root cause(s) before establishing that the data point truly is not comparable. In addition to cell culture process performance indicators, critical quality attributes (CQAs) are analyzed for a purified product collected from qualification runs. The TOST statistical approach can be used to evaluate CQAs, but a simpler approach may be applied comparing CQAs of material generated from the scale-down model to the acceptance ranges and criteria established for the large-scale product.
The large-scale process for our scale-down model described herein uses adherent cells grown on microcarriers. Several publications have reported microcarrier culture sensitivity to shear stress and microcarrier collision in a stirred tank (16–18). Shear stress can lead to cell detachment from microcarriers, which can ultimately affect the performance of a culture. Although agitation is a source of shear stress, a minimum agitation rate is required to keep microcarriers suspended in a bioreactor. The amount of shear stress to which cells are exposed at small and large scales probably would be different because of differences in agitation rates, design and geometry, gas-sparging strategy, the number of baffles present in each bioreactor with respect to vessel size, and geometry of the vessel. Process engineers need to consider these factors when developing a scale-down model that supports a microcarrier culture.
The microcarrier culture described herein is an existing commercial manufacturing process. The approach we used to develop a scale-down model for an existing process is similar to that followed for a new process except for the availability of a large data set at manufacturing scale. Those data can be used to compare performance of the scale-down model in development with the large-scale process and ultimately to increase confidence in data generated from the model.
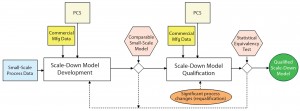
Figure 1: Approach for developing and qualifying scale-down models for large-scale processes (adapted from an internal company document); PCS = process control strategy.
Figure 1 illustrates our overall strategy for development and qualification of scale-down models of existing commercial manufacturing processes for upstream, downstream, and drug-product lyophilization processing. The large-scale process control strategy (PCS) is adapted during scale-down model development and qualification. Commercial manufacturing data also are included to refine the model during development. That same set of commercial manufacturing data is used during qualification to determine statistical equivalency for the scale-down model. Redevelopment of such a model usually is unnecessary unless significant changes are made to a manufacturing process. Once the scale-down model is qualified, it is a very powerful tool to use for manufacturing-deviation investigations, for furthering process characterization, and in future process improvement as part of product life-cycle management.
Materials and Methods
Cell Culture: We propagated Chinese hamster ovary (CHO) cells that express a recombinant therapeutic enzyme on macroporous microcarriers in 15-L stirred-tank Broadley James bioreactors with a 12-L working volume. These cultures operated in perfusion mode for about 65 days. After a short period of growth in batch mode immediately following inoculation, the system fed medium continuously into the bioreactors to provide nutrients, with harvest fluid removed using a proprietary cell separation device to collect the product of interest and to reduce metabolic waste accumulation (19). The cell-separation device uses gravity to separate microcarriers (with cells attached) from supernatant.
We used two different proprietary Genzyme media sequentially to provide nutrients (e.g., amino acids and vitamins) to the cultures. One medium type was used for the culture’s five day growth phase during which cells reached a targeted density for production. We used a second medium type for the remainder of the culture while harvesting product from the bioreactors (about 60 days). Product was harvested continuously into collection vessels before downstream processing. Parameters such as temperature, pH, and dissolved oxygen (DO) were monitored and controlled to a target set-point.
Culture pH was maintained within a desired operating range using pH probes (Broadley James) that were calibrated against a daily offline pH measurement using a Bayer Rapidlab 248 blood gas analyzer as needed. Dissolved oxygen probes (Broadley James) were used to maintain the DO set point at 50%, and the resulting oxygen sparge rate served as a secondary indicator for cell growth. Additionally, we used pCO2 probes (YSI Life Sciences) to monitor the cultures and target the pCO2 range at large scale. A DeltaV automation system (Emerson Process Management) with feedback control capability monitored and controlled pH, DO, temperature, and gases. We set up perfusion control (continuous feed and harvest) through the DeltaV system. All these parameters were matched to the large scale, with the exception of bioreactor vessel, impeller size, cell-separation device size, and harvest-collection vessel size.
Analytical Methods: We assessed cell growth, metabolism, and productivity through offline measurements. A ViCell XR (Beckman Coulter) measured cell density and viability. We treated cell culture samples collected from the bioreactors with an enzyme to detach cells from their microcarriers before obtaining cell counts. A YSI 2700 metabolite analyzer generated cellular metabolic profiles for glucose, lactate, glutamine, and glutamate. We measured protein productivity using a photometric enzymatic activity assay. And appropriate analytical tools were used to assess drug-substance material purified with a qualified scale-down purification model for select CQAs such as specific activity, glycosylation profile, and charge variants.
Model Development: Figure 2 illustrates our overall approach for developing a scale-down model. Because of the reduced volume of the 12-L bioreactor, we applied minor adjustments to the seed-train process. Although it was possible to keep the same vessel sizes for most seed-train passages, we reduced the N – 1 vessel size to accommodate the scale-down bioreactor. But we kept all other parameters associated with the seed train (e.g., the number of passages and scale-up criteria) identical to those of the large scale.
In addition to the N – 1 seed-train and production vessels, we scaled down the size of the cell-separation device accordingly. Both it and the bioreactor vessel are made of glass in the scale-down model, whereas the large-scale equipment is stainless steel. For harvest collection, we use plastic bags at the small scale, whereas stainless steel vessels are used at the large scale.
An additional physical difference between the two scales is the impeller configuration. The 12-L scale uses dual elephant-ear impellers; the 2,000-L bioreactor is equipped with a single elephant-ear impeller. Dual impellers in the scale-down model address a difference in the ratios of liquid height to tank diameter between the two scales. Furthermore, the impeller diameter ratio between the 2,000-L and 12-L bioreactors is about 10:1, and the ratio of impeller to tank diameter between the two scales is about 1.6:1.
During model development, we studied two primary parameters: agitation rate and sparger configuration. Based on either P/V or tip-speed matches to the large-scale process, we evaluated several agitation rates to determine an optimal rate for comparable cell culture performance. Controlling pCO2 within the range established at full scale is critical because elevated levels can affect cell culture performance (20). During development of our large-scale process, high pCO2 levels had been shown to affect cell culture performance.
To align the pCO2 levels between the two scales, we evaluated several sparger configurations. First, we tested a single sparger element to supply both oxygen to cells and nitrogen to strip CO2 from the culture (to maintain pCO2 within the desired range). But it was difficult to control CO2 levels with a single sparger element because of competing gas flow between oxygen and nitrogen. So we later evaluated a dual-sparger configuration that matched the large-scale configuration, in which two sparger elements provide independent oxygen and nitrogen gas to the culture.
Other process control parameters in the small-scale model followed the large-scale process according to its process control strategy. We then compared data generated with the small-scale model with data from the large-scale process to assess whether a certain parameter required further optimization.
Model Qualification: We conducted a qualification study after successfully developing our 12-L scale-down model. We considered the model to be fully developed when a minimum of three small-scale runs demonstrated comparable performance to the largescale process in terms of growth, productivity, and metabolism. To calculate the number of runs required to qualify the scale-down model statistically, we used this sample-size equation (21):
where N = number of runs; α = confidence level (α = 0.1 means 90% confidence level); 1 – β = power; Z = inverse of cumulative standard normal distribution function; k = number of standard deviations. This calculation was based on two standard deviations of the large scale: a power number of 0.9 (β = 0.1) and a confidence level of 90%. Based on that equation, we determined that five runs would be required to demonstrate equivalency to the large scale within two standard deviations.
Next, we executed an experiment of five bioreactor runs based on the description provided in the “cell culture†section above. The cultures were sampled daily for the first 10 days, then three times each week for the remaining 55 days. To determine equivalency, we compared data obtained on sampling days statistically with information from the large scale, monitoring five cell culture parameters that represented cell growth, metabolism, and productivity: viable cell density (VCD), glucose concentration, lactate concentration, the yield of lactate from glucose (as a ratio), and volumetric production rate (VPR). Those five parameters are the most critical indicators representing performance of a culture.
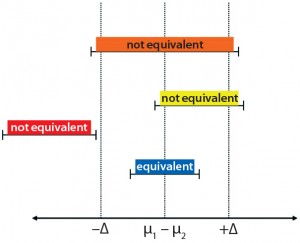
Figure 3: Datasets considered to be equivalent (blue bar) and nonequivalent (red, orange, and yellow bars); when the difference between group means are within predefined tolerability limits (±Δ), the datasets are considered to be statistically equivalent.
Statistical Analysis: We applied a TOST equivalency analysis for qualification of our 12-L scale-down model. It is an established statistical tool used for determining statistical equivalency between two data sets (13–15). TOST analysis was performed on all sampling days to determine whether data generated from the 12-L scale were statistically equivalent to the large scale. We used a predefined tolerability range (–Δ, Δ) to determine whether the two sets of data were comparable, with a tolerance limit of two standard deviations based on internal company guidance. Figure 3 illustrates possible equivalency results.
Datasets are determined to be statistically equivalent when 90% confidence intervals of the difference in group means (μ1 – μ2) are within the tolerability limit (±Δ). A justification is needed when more than three consecutive failure points come up during qualification. Otherwise, a parameter is deemed to be nonequivalent (22).
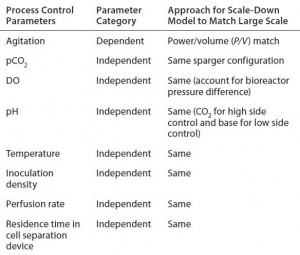
Table 1: Summary of scale-dependent and scale-independent parameters considered during development of the 12-L scale-down model
Results and Discussion
Development of the 12-L Scale-Down Model: Table 1 summarizes the scale-dependent and scale-independent parameters we considered during development of our 12-L scale-down model. Scale-independent variables — DO, pH, temperature, and inoculation density — were all operated to match those of the large scale. We did not directly control the pCO2 level, another scale-independent variable. More comprehensive studies were needed to evaluate different nitrogen sparger configurations and gassing strategies to target the pCO2 ranges of the large scale (detailed below). Additional scale-independent parameters more specific to a perfusion cell culture process include perfusion rate and culture residence time in the cell-separation device. We set those identically to those of the large scale by designing a small-scale cell-retention device that matched those important perfusion parameters. We also used the same media at both scales. Agitation rate (a scale-dependent variable) was evaluated extensively, and the results are discussed in detail below.
Optimization of Parameters — Agitation: Agitation rate was broadly evaluated during development of this 12-L scale-down model. Because vessel size and geometry (e.g., height:diameter ratio) are different between the small and large scales, we had to determine an optimal agitation rate to keep microcarriers suspended in culture, to provide adequate oxygen transfer to cells, and to get growth characteristics similar to those of the large scale. Because this cell line is shear sensitive (like most microcarrier cultures in general), we tested a number of agitation strategies including conventional scale-up/scale-down approaches such as matching tip speed or P/V as well as applying a more conservative approach of testing minimal agitation rates needed to keep microcarriers suspended in the bioreactor (6).
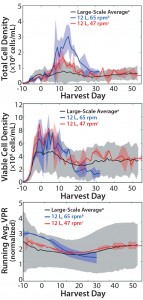
Figure 4: (top) Suspension total cell density, (center) viable cell density (VCD), and (bottom) normalized running-average volumetric production rate (VPR) for the large-scale average (n = 33), 12-L 65-rpm average (n = 2), and 12-L 47-rpm average (n = 3); the 65-rpm 12-L runs were terminated early for poor culture performance. Normalized runningaverage VPR profiles were normalized to the maximum average of the large scale for each respective parameter. Shaded areas represent two standard deviations from the mean for all three parameters.
We evaluated four agitation rates at the 12-L scale, including 65 rpm (tip speed match to the large scale), 47 rpm (P/V match to the large scale), and 24 rpm and 32 rpm (determined to represent the lower boundary for the 12-L model). Operation at 24 rpm allowed a significant amount of cells to settle in the 12-L bioreactor, indicating insufficient mixing to keep the microcarriers suspended in culture. We observed a nonhomogeneous culture with large aggregates of cells and microcarriers at 32 rpm, eliminating that as a possible agitation rate for the 12-L scale as well. Both higher agitation rates we tested yielded well-suspended cultures, so we compared the corresponding cell growth profiles to determine the best agitation rate for the small scale. Operation at 65 rpm (tip-speed match) caused an increase in the number of suspension cells that had detached from their microcarriers, probably a result of increased shear stress. Finally, we determined 47 rpm (P/V match) to be the appropriate agitation rate at the 12-L scale for providing adequate oxygen transfer, sufficient microcarrier suspension, and comparable growth and productivity characteristics to the large scale (Figure 4). P/V is typically the appropriate scaling parameter for microcarrier cultures (17).
Optimization of Parameters — pCO2 Level: Although pCO2 level is categorized as a scale-independent variable, it is not a parameter that can be controlled directly, but instead must be manipulated through a gassing strategy. To match the pCO2 target ranges established for the large-scale process, we studied the mechanical configuration of oxygen and nitrogen sparger(s) to determine the optimal strategy for the 12-L scale-down model.
First, we evaluated a 5-μm single sintered sparger element to provide both oxygen and nitrogen gases to the culture for maintaining DO levels and stripping CO2 from the culture. As Figure 5 shows, the single sparger element allowed for an elevated CO2 level that was beyond the large-scale range. Subsequently, we evaluated two sintered sparger elements to match the large-scale configuration and provide independent oxygen and nitrogen sparging to the culture.
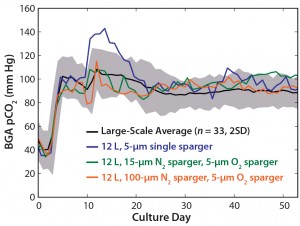
Figure 5: BGA pCO2 profiles represent the large-scale average (n = 33); a 12-L model with a 5-μm single sparger; a 12-L model with a 15-μm N2 sparger and a 5-μm O2 sparger; and a 12-L model with a 100-μm N2 sparger and a 5-μm O2 sparger. Shaded areas represent two standard deviations from the mean.
For nitrogen sparging, we tested two pore sizes (15 μm and 100 μm) to maintain pCO2 and oxygen sparge profiles that would be similar to those of the large scale. For oxygen sparging, we conducted mass-transfer studies to understand the oxygen-transfer dynamics in the 12-L model, determining that a sparger element with a 5-μm pore size yielded volumetric oxygen sparge profiles similar to those of the large-scale bioreactor.
Figure 5 also illustrates the effects of a dual-sparger configuration and different nitrogen sparger pore sizes on the pCO2 profile. Results suggested that independent delivery of oxygen and nitrogen could match the pCO2 ranges of the large scale regardless of nitrogen sparger pore size. Between the two pore sizes, we chose the 100-μm nitrogen sparger pore size for the model because it provided more efficient CO2 removal from the culture (23). That sparger element reduced the nitrogen sparge flow rate and total foam level on the liquid surface, which at higher levels can negatively affect cell cultures.
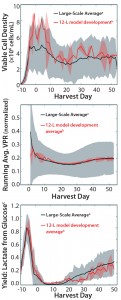
Figure 6: (top) Viable cell density (VCD), (center) normalized running average volumetric production rate (VPR), and (bottom) yield of lactate from glucose profiles for developed 12-L scale-down model average (n = 3) and largescale average (n = 33); the 12-L model development averages were normalized to the maximum value of the large-scale average for each respective parameter. Shaded areas represent two standard deviations from the mean for all three parameters.
Model Development Results: Figure 6 profiles VCD, average VPR, and yield of lactate from glucose ratio for representative and successful development runs. We normalized the average VPR and yield of lactate from glucose profiles to the maximum value of the large-scale average calculated within the course of the culture. The red-shaded area for the 12-L development runs and the grey-shaded area for the large-scale runs represent two standard deviations from their respective means. The cell culture parameters shown reflect the two phases of the perfusion culture process.
Cells grew for about five days before another five days of a transition period, during which production medium was perfused into the bioreactor. After that, the harvest phase began, and product was collected for downstream processing. The production medium is “leaner†than the growth medium, which explains the dynamic cell culture profiles observed as the culture moved from growth through the transition and harvest phases. Results of our development studies confirmed that implementing an agitation rate of 47 rpm and a second sparger element with a 100-μm pore size for nitrogen sparging in the 12-L scale-down model yielded growth, productivity, and metabolic profiles similar to those of the large-scale process.
Qualification of the 12-L Scale-Down Model: Successful scale-down model qualification requires key attributes in at least five complete 12-L bioreactor runs to be statistically equivalent to those of relevant large-scale bioreactor runs. To qualify the cell culture scale-down model, we assessed cell culture growth, metabolism, and productivity to determine equivalency using TOST analysis. We also assessed product quality of purified material based on the acceptance criteria for different characterization parameters. Five concurrent bioreactor runs were conducted for the qualification study.
Cell Culture Results: Figure 7 shows three of the five cell-culture parameters evaluated for demonstration of statistical equivalency. In each graph, the largescale tolerance range is normalized to +1 (maximum) and –1 (minimum) on the y-axis — represented by the grey shaded area. Each data point is also normalized respectively and represents the scaled difference between the averages of the small-scale (12-L qualification runs) and large-scale runs on each sampling day. Error bars represent the 90% confidence interval of the difference in group means between the large scale and the 12-L scale each day.
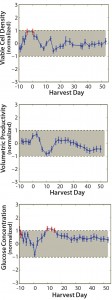
Figure 7: Normalized (top) viable cell density (VCD), (center) volumetric production rate (VPR), and (bottom) glucose concentration profiles for the 12-L qualification average (n = 5, 90% confidence interval, CI) and the acceptable tolerance range; profiles were normalized to the large-scale tolerance range represented by +1/–1 on the y-axis and the grey shaded area. Each data point represents the scaled difference between the 12-L small-scale and large-scale means, and error bars represent 90% confidence intervals of the difference between the two group means. Red data points represent data that fell outside the tolerance limits.
In Figure 7 (top), the VCD profile shows two consecutive data points in red that did not meet acceptance criteria. As mentioned above, we had deemed it acceptable to have a maximum of three consecutive failures during qualification of the scale-down model. Therefore, the two consecutive VCD failures remain within our qualification acceptance criteria.
The VPR profile in Figure 7 (center) demonstrates that all small-scale data points fell within the tolerance interval. That successfully establishes their equivalency with the large-scale data.
Figure 7 (bottom) shows the glucose concentration profile. Three consecutive glucose concentration data points fell outside the tolerance limit, indicated by the red data points. However, we considered that to be acceptable based on the “maximum-of-three†parameter mentioned above.
Our evaluations of lactate concentration and yield of lactate from glucose also demonstrated statistical equivalency (data not shown). Data show that all parameters successfully established equivalency between the large-scale process and scale-down model.
Product Quality Results: Protein product was collected from the five 12-L bioreactors throughout the harvest phase. Harvested fluid collections were pooled similarly to the approach established for the large-scale process. Each representative small-scale harvest collection included three to four days of harvest material collected from early, middle, and late culture. The harvest materials were combined using a strategy similar to that of the large-scale process and purified to produce drug substance (DS) material. We compared that material with large-scale historical ranges and against product-release acceptance criteria, evaluating all product quality attributes analyzed for large-scale DS lot release for the DS material derived from our five 12-L qualification bioreactors. Figure 8 shows an example in which five CQAs from the small-scale DS material fell within the large-scale acceptance criteria.
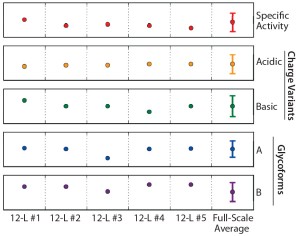
Figure 8: Normalized critical quality attribute (CQA) data for purified material derived from each 12-L qualification run (n = 5); error bars on the large-scale data represent acceptance criteria for each particular product quality attribute.
Application of the Scale-Down Model
Developing a qualified scale-down model establishes the ability to predict large-scale performance based on results from experiments conducted with the model. Applications for such models include exploring raw materials (e.g., evaluating the impact of medium additives on cell culture performance, qualifying critical raw materials) and supporting large-scale process troubleshooting and deviation investigations (e.g., qualification of new GMP cell banks before implementing them at large scale). A qualified scale-down model also can be used to build more knowledge about varying process control parameters on cell culture performance for further process characterization (1, 2).
The limitations of scale-down models should be considered. Not all scale-down model process parameters can match those at large scale, so careful consideration should be made when designing studies to support the large scale. Some limitations relating to vessel geometry differences and shear stress would be difficult to match simply due to scale differences. Despite attempts to use different conventional scaling strategies such as P/V or tip speed match to scale-down agitation rate, the agitation rate selected for a scale-down system may not reproduce the same hydrodynamic and mixing characteristics of the large-scale system, which may cause varying turbulence patterns in the bioreactor (3).
Gas sparging/aeration is another major parameter that is difficult to match between large- and small-scale models. Because of different liquid surface-to-volume ratios, CO2 stripping is usually much more efficient in small-scale models. That creates different nitrogen sparging requirements to match pCO2 levels between the two scales. Gas bubble residence times in a bioreactor also differ between large and small scales because of different bioreactor geometries.
Because of the different sparging requirements and agitation strategies in scale-down models, shear stresses experienced by cells typically differ between small and large scales. That may yield different culture performance for shear-sensitive cell lines. For those studying mixing characteristics and the impact of gas sparging and shear stress on a given system, computational fluid dynamics can be an important tool to help bridge the gap between the small and large scales.
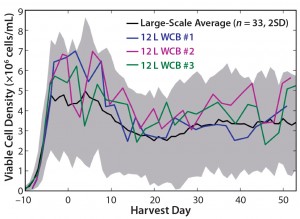
Figure 9: Viable cell density (VCD) profiles for the large-scale average (n = 33), 12-L working cell bank (WCB) #1, 12-L WCB #2, and 12-L WCB #3; shaded area for the large-scale average represents two standard deviations from the mean.
Evaluation of New Working Cell Banks (WCBs): One application for which our qualified scale-down model has been used is in evaluation of new WCBs before large-scale implementation. We evaluated three new WCBs using the qualified 12-L scale-down model. Figure 9 shows VCD profiles for those three WCBs. The growth profile for each new bank was comparable to that of the large scale, as were other parameters not shown here, including productivity and metabolism. Applying the qualified model for new WCB evaluations can ensure that a newly generated GMP WCB will perform as expected before implementation at the large scale.
A Working Model
We successfully developed a 12-L scale-down model to match cell culture performance at the 2,000-L scale. The developed model was subsequently qualified by demonstrating statistical equivalency with the large-scale process for five key cell culture performance indicators related to cell growth, productivity, and metabolism. All product quality attributes were comparable and within acceptance ranges. Although statistical equivalency was established for both scales, limitations of a 12-L qualified scale-down model should be considered when using such a model to conduct manufacturing support studies. Our qualified model has since been used for evaluation of new WCBs for manufacturing implementation and will continue to provide increased confidence in data generated for future characterization studies and support.
Acknowledgments
The authors thank the following groups and people at Genzyme, a Sanofi Company: cell banking development, process analytics, commercial cell culture development, scaledown model development and qualification practices teams, statistics; and Julie Barker, Rakesh Motani, Ashwini Ranjan, Hang Zhou, Benjamin Wright, Jesse Keegan, Claudia Buser, and Veena Warikoo.
References
1 Seeley JE, Seely RJ. A Rational, StepWise Approach to Process Characterization. BioPharm Int. 16(8) 2003: 24–34.
2 Li F, et al. Cell Culture Processes for Monoclonal Antibody Production. MAbs 2(5) 2010: 466–477.
3 Venkat RV, Chalmers JJ. Characterization of Agitation Environments in 250-mL Spinner Vessel, 3-L and 20-L Reactor Vessels Used for Animal Cell Microcarrier Culture. Cytotechnol. 22, 1996: 95–102
4 Michaels JD, Mallik AK, Papoutsakis ET. Sparging and Agitation-Induced Injury of Cultured Animal Cells: Do Cell-to-Bubble Interactions in the Bulk Liquid Injure Cells? Biotechnol. Bioeng. 51(4) 1996: 399–409.
5 Chisti Y. Animal-Cell Damage in Sparged Bioreactors. Trends Biotechnol. 18(10) 2000: 420–432.
6 Rao DG. Introduction to Biochemical Engineering (2nd Edition). Tata McGraw Hill: New Delhi, India: 2010.
7 Kelley WJ. Using Computational Fluid Dynamics to Characterize and Improve Bioreactor Performance. Biotechnol. Appl. Biochem. 49(4) 2008: 225–238.
8 Fang Z. Applying Computational Fluid Dynamics Technology in Bioprocesses, Part 2: Computational Fluid Dynamics Can Resolve Performance Problems. BioPharm Int. 23(5) 2010: 42–52.
9 Bezzo F, Macchietto S, Pantelides CC. General Hybrid Multizonal/CFD Approach for Bioreactor Modeling. AIChE J. 49(8) 2003: 2133–2148.
10 Julien C, Whitford W. Bioreactor Monitoring, Modeling, and Simulation. BioProcess Int. 5(1) 2007: S10–S17.
11 Alam MNHZ, Razali F. Scale-Up of Stirred and Aerated Bioengineering Bioreactor Based on Constant Mass Transfer Coefficient. J. Teknol. 43(F) 2005: 95–110.
12 Garcia-Ochoa F, Gomez E. Bioreactor Scale-Up and Oxygen Transfer Rate in Microbial Processes: An Overview. Biotechnol. Adv. 27, 2009: 153–176.
13 Li F, et al. A Systematic Approach for Scale-Down Model Development and Characterization of Commercial Cell Culture Processes. Biotechnol. Progr. 22(3) 2006: 696–703.
14 Chen C, et al. Statistical Equivalence Testing for Assessing Bench-Scale Cleanability: The Two-One-Sided T-Test Compares the Equivalency of Two Data Sets. BioPharm Int. 23(2) 2010: 40–45.
15 Barker LE, et al. Assessing Equivalance: An Alternative to the Use of Difference Tests for Measuring Disparities in Vaccination Coverage. Am. J. Epidemiol. 156(11) 2002: 1056–1061.
16 Cherry RS, Papoutsakis ET. Physical Mechanisms of Cell Damage in Microcarrier Cell Culture Bioreactors. Biotechnol. Bioeng. 32(8) 1988: 1001–1014.
17 Croughan MS, Hamel JF, Wang DIC. Hydrodynamic Effects on Animal Cells Grown in Microcarrier Cultures. Biotechnol. Bioeng. 95(2) 2006: 295–305.
18 Hu WS. Quantitative and Mechanistic Analysis of Mammalian Cell Cultivation on Microcarriers (doctoral dissertation). Massachusetts Institute of Technology: Cambridge, MA, 1983.
19 Barngrover D, et al. Continuous Settling Apparatus. US Patent 5,733,776; 31 March 1998.
20 Zhu M, et al. Effects of Elevated pCO2 and Osmolality on Growth of CHO Cells and Production of Antibody-Fusion Protein B1: A Case Study. Biotechnol. Progr. 21(1) 2005: 70–77.
21 Chow S, Shao J, Wang H. Sample Size Calculations in Clinical Research (2nd Edition). Chapman and Hall/CRC Press: Boca Raton, FL, 4 March 2003.
22 Western Electric Company. Statistical Quality Control Handbook (2nd Edition). Mack Printing Company: Easton, PA, 1958; www.contesolutions.com/Western_Electric_SQC_ Handbook.pdf.
23 Gray DR, et al. CO2 in Large-Scale and High-Density CHO Cell Perfusion Culture. Cytotechnol. 22(1–3) 1996: 65–78.
Corresponding author Caroline DiCesare is a process engineer II in manufacturing science and technology (Framingham Biologics, 68 New York Avenue, Framingham, MA 01701; caroline.dicesare@ genzyme.com); Marcella Yu is a staff scientist II, Jin Yin is associate director, and Chris Hwang is senior director of commercial cell culture development; Jennifer Tengtrakool is manager of manufacturing science and technology, and Konstantin Konstantinov is vice president of late-stage process development; all at Genzyme, a Sanofi Company. Previously a member of their team, Weichang Zhou is now senior vice president/biologics development and manufacturing at WuXi AppTec Co., Ltd., 288 Fute Zong Road, Shanghai 200131, China.