The analytical program for a given biotherapeutic has a life-cycle analogous to that of a manufacturing process used to prepare material for clinical and commercial use. This two-part article discusses analytical activities associated with the progression of biotherapeutic candidates from the early stages of clinical development through their appearance as licensed drugs on the market. In Part One, we examined the stages of the analytical life-cycle. Here we conclude by going into more detail on challenges associated with method qualification, validation, and remediation.
Important Concepts in Method Qualification and ValidationMethod qualification (MQ) has frequently been viewed as a “box-checking” exercise with no inherent value other than in nonrigorously demonstrating an analytical method’s suitability for its application. In many cases, qualification has been approached without target expectations regarding method performance. We advocate the establishment of target expectations for qualification of various method types. These expectations should be based on prior experience with methods and technologies used for evaluating a given quality attribute. In the absence of target expectations, it is very difficult to assess whether a method indeed has desired capabilities, and method developers have no targets that can demonstrate the success of their efforts when achieved. In addition, the lack of target expectations can generate significant differences in performance of the same methods when they are applied to different products. Later in development (but before method validation), these expectations take on a more formalized character as acceptance criteria.
Data Reporting: Another rationale for performing qualification before method execution in a quality environment is that data reporting intervals must be established to ensure their alignment with the method’s capability. One common way of establishing a reporting interval is to derive it from method precision (7). Recently Agut et al. examined different rules and their application to reporting intervals of results and specifications (8). The best known and simplest rule is that stated in the ASTM standard E-29-02, which states that analytical measurement results should be rounded to no less than 1/20 of the determined standard deviation (9).
For example, bioassays with a 12.8 standard deviation should adopt a reporting interval larger than 0.64. However, 0.64 is impractical in day-to-day applications. Therefore, bioassays with a 12.8 standard deviation would have a reporting interval of 1.0. Similarly, an HPLC assay with a 1.1. standard deviation for its main peak would have to adopt a reporting interval of 0.1. Reporting intervals for impurities need to be consistent with reporting intervals for the main peak. Table 3 recommends the nearest reporting intervals based on standard deviations obtained during qualification.
Table 3: Recommended nearest reporting intervals in relationship to method precision
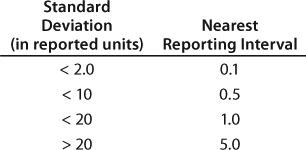
Table 3:
Target Expectation for Precision: Method precision is closely linked to the concentration of an analyte. Therefore, qualification precision expectations for analytical methods may vary from analyte to analyte. As the analyte (component) content decreases, eliminating interference from the sample matrix becomes increasingly difficult.
The target value for the RSD of measurements can be established in advance. The best-known relationship between analyte concentration and RSD comes from the Horwitz equation (2,3,4,5):
In our design, the target for intermediate precision is typically two-thirds of the %RSD derived from the Horwitz equation. Thus, intermediate precision studies conducted during qualification should meet two-thirds of the variability derived from the equation for each individual analyte. For method qualification, the targets for intermediate precision and repeatability are set at the same value. During the qualification experiments, if the measurement of precision exceeds values derived from the Horwitz equation, then an assay may need to be redeveloped or its technology may not be fully suitable for the intended application.
Typically, proteins are available for analysis in solution, with their concentrations ranging widely from 1 mg/mL (e.g., a growth factor) to 100 mg/mL (e.g., a monoclonal antibody, MAb). Expectations for the RSD of measurements for the main protein analyte in such solutions would be 16% and 2.8%, respectively. Table 4 below delineates intermediate precision targets with respect to analyte concentration.
Table 4: Target expectations of precision derived from Horwitz equation relative to analyte concentration
Assessment of the limits of detection and quantification (LOD and LOQ) is required for most analytical methods developed to monitor product quality attributes. ICH defines LOD as the minimum level of analyte that can be readily detected, and LOQ has been defined as the minimum level that can be quantified with acceptable accuracy and precision. Practical application of LOD is related to decisions made about integration of chromatograms, electropherograms, or spectra. LOQ is related to decisions made regarding whether to report test results on official documents such as the certificate of analysis (C of A) for a given lot.
ICH Q2R1 suggests three different approaches (1): visual inspection, signal-to-noise-ratio, and variability of the slope of a calibration curve (statistical approach, see below). Vial and Jardy evaluated different approaches for determining LOD/LOQ and concluded that they generate similar results (10). A similar conclusion was reached by Miller at al. (11). It is prudent to verify LOD/LOQ values obtained using different calculations. If those values are not within the same order of magnitude, than the integrity of their source data should be investigated.
The statistical approach is most commonly practiced and is associated with the use of two well known equations:
The slope used in such equations is equivalent to instrument sensitivity for a specific analyte, reinforcing the fact that LOD and LOQ are expressed in units of analyte concentration (e.g., mg/mL) or amount (e.g., mg). Because they are functions of instrument sensitivity, these values, when defined thusly, are not universal properties of a method that would be transferable from instrument to instrument or analyte to analyte.
LOD is essential when deciding to include or disregard a peak for integration purposes stresses the importance of signal-to-noise ratio as a key parameter governing peak detection. Defining LOD as 3.3 × noise creates a detection limit that can serve as a universal property of methods applicable to all analytes and different instruments (because the instrument sensitivity factor has been disregarded). LOD expressed thusly is a dynamic property that depends on the type/status of instrument and quality of consumables. LOD determined this way will be expressed in units of peak height (e.g. mV or mAU).
Deciding whether to report a specific analyte on a C of A is typically linked to specifications. After a decision has been made about integration for all analytes resolved (defined) by a given method, the results are recorded in a database (e.g., LIMS). When all analytical tests are complete, the manufacturer creates a C of A by extracting relevant information from that database. Only a subset of the results (defined by specifications) will be listed. Specifications will depend on the extent of peak characterization and the clinical significance of various peaks (12). So the list will change (evolve) throughout drug development. In such a context, LOQ should be considered as the analyte-specific value expressed in units of protein concentration, a calculation for which instrument sensitivity cannot be disregarded (by contrast with LOD estimation). Therefore, a potential exists for diverse approaches to determining both LOD and LOQ.
Application of LOD/LOQ to purity methods presents specific challenges that deserve additional consideration. The reporting unit is percent (%) purity, a unit that is incompatible with the units typically used to express LOD and LOQ (concentration or amount). The signal created by an analyte may vary with its load, whereas its relative percentage does not change. This creates a situation in which a peak of interest can be hidden by noise or alternatively the peak may be expressed significantly above the noise for the same sample analyzed at two different load levels within the method’s range. This situation creates an environment for misinterpretation of test results and confusion among analysts conducting the testing. Expressing LOD in units of peak height can significantly alleviate the issue.
Method Development Reports: The development and qualification history of analytical methods intended for a GMP environment should be documented in a method development report (MDR). This preserves the intellectual property rights inherent in method design and provides “institutional memory” if the development scientist is unavailable to provide technical input for future practitioners of the method and/or responses to regulatory questions.
It is advisable to have an MDR template to ensure that compliance needs are consistently met and to streamline the process of drafting such documents. A specific report might not contain the same content in all the sections. In such cases, the template should not be altered; unaddressed sections should reference previous work, state that such work will be conducted in the future, or note that the work for that section is irrelevant for the given circumstances. If new information is obtained, then compliance needs dictate that it should be put in an addendum to the MDR rather than revising the original document, so the original content is preserved.
Method RemediationRemediation of analytical methods is typically triggered by a need to improve existing methods used for commercial products. An improvement may be required due to frequent method failures in the GMP environment, lengthy run times, obsolete instruments or consumables, the changing regulatory environment for specifications or stability testing, or other business reasons.
Frequently, old methods must be replaced with newer technologies, which creates significant challenge for the industry. On one hand companies are encouraged to pursue innovative approaches, such as process analytical technologies (PAT); on the other hand, regulatory requirements for implementing technological improvements for commercial products are burdensome and complex.
In the electronics industry, Moore’s Law is a well-established paradigm that describes the progress of electronic processing speed. It dictates that the capacity of microelectronic systems (microchips) to process information doubles every 18 months. If we apply such an analysis to the separation sciences, we can estimate their rate of progress. For example, Figure 2 depicts the historical run time of amino acid analysis. Steady progress has been made in improving run times for amino acid analysis, with a generation time of ∼11 years, defined by the time it takes to double the method throughput (reduce the runtime two-fold). We selected amino acid analysis because of the existing long-term literature available and the fact that its objective — resolution of 20 amino acids — is intrinsically embedded into its run time. We believe this analysis may approximate the progression of separations techniques and perhaps other analytical disciplines as well.
The advent of microfluidic technologies (e.g., the recently developed chip-based separation technology from Caliper Technologies, www.caliperls.com), promises to further advance the separation sciences. Recently, it has become possible to use such new technology to separate the heavy and light chains of MAbs in 30 seconds, a significant leap forward in runtime relative to the typical 30-minute run-time of capillary electrophoresis separations. We anticipate that such technological advances will continue to drive the separation sciences toward increasing throughput.
In many cases, release methods are destined for change as soon as a product has been approved for commercial use. It takes >10 years to commercialize a biotechnology drug, resulting in significant aging of analytical methods developed at the conception of a given project. Regulators and the industry will need to continuously adjust their strategies to address this issue of old and new methods, particularly with respect to how these advances affect product specifications (12).
Concluding Remarks: When we consider the critical roles analytical method development, qualification, and validation play in the biopharmaceutical industry, the importance of a well-designed strategy for the myriad analytical activities involved in the development and commercial production of biotech products becomes evident. Our analytical life-cycle strategy provides a framework and can be viewed as a modular design encompassing distinct activities with deliverables that cover the entire product development life-cycle. Because of this modular design, the significant workload inherent in these various activities can be divided among different parts of a corporate organization.
Thus, multiple design scenarios can be envisioned in which analytical activities can be carried out in by research, development and/or quality groups, with no single design model of the “right” way to go about those activities. It is therefore possible to fulfill these needs in most if not all organizational designs throughout the industry. Finally, organizing the work this way provides a scaleable approach to developing multiple product candidates simultaneously, with iterative learning providing a basis for improving performance for ongoing and future projects.
Method qualification activities provide a strong scientific foundation during which the performance characteristics of a method can be assessed relative to preestablished target expectations. This strong scientific foundation is key to long-term high performance in a quality environment following method validation, which serves as a critical pivotal point in the product development life-cycle. As noted, method validation often serves as the point at which a quality group assumes full “ownership” of analytical activities. This contributes to operational excellence as evidenced by low method failure rates, a key expectation that must be met to guarantee organizational success. Without a strong scientific foundation provided by successful method development and qualification, it is unlikely that operational excellence can be achieved in the quality environment.
Establishment of qualification target expectations can be considered a form of quality by design (QbD) because this methodology establishes quality expectations for a method before its development is complete. Also, the analytical life-cycle we describe covers all aspects of method progression, including development, qualification, and robustness at both the early and late stages of product development, as well as method validation, which establishes that a method is suitable for the quality laboratory environment. The entire analytical life-cycle framework can, in fact, be considered as a QbD process. As analytical technologies continue to evolve, both the biotechnology industry and its governing regulatory authorities will need to continuously develop concepts and strategies to address how new technologies affect the way QbD principles inherent in the analytical life-cycle approach are applied to the development of biopharmaceutical products.